
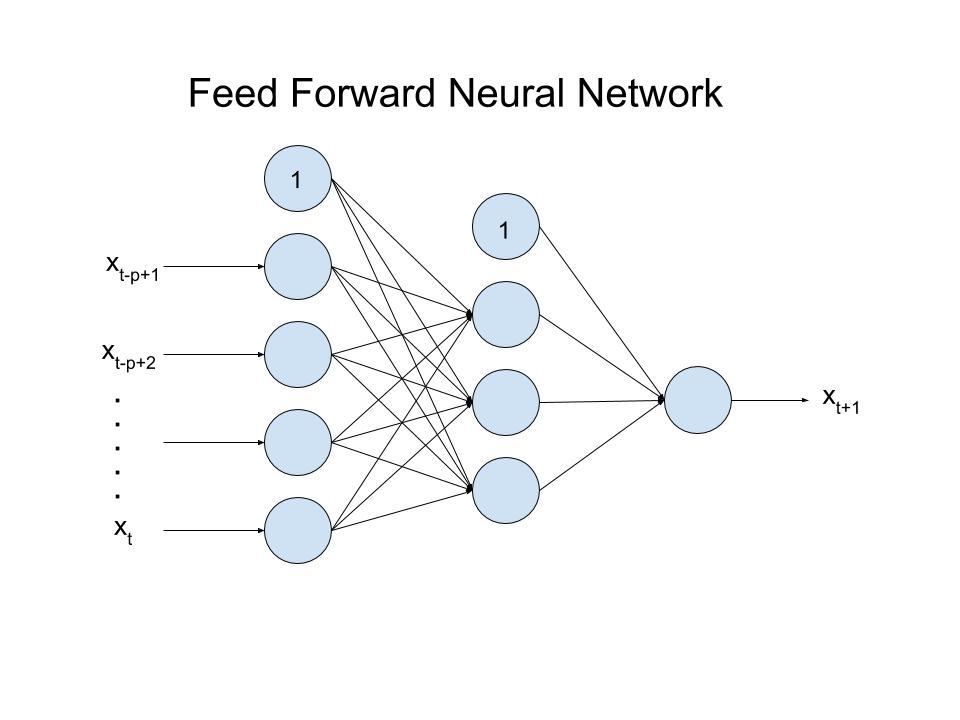
To the best of authors’ knowledge, this article improves the state-of-the-art for high-performance yet low-complexity radar detection in critical indoor sensing applications.Ībstract: Target detection is a mandatory task of radar system so that the radar system performance is mainly determined by its detection rate.
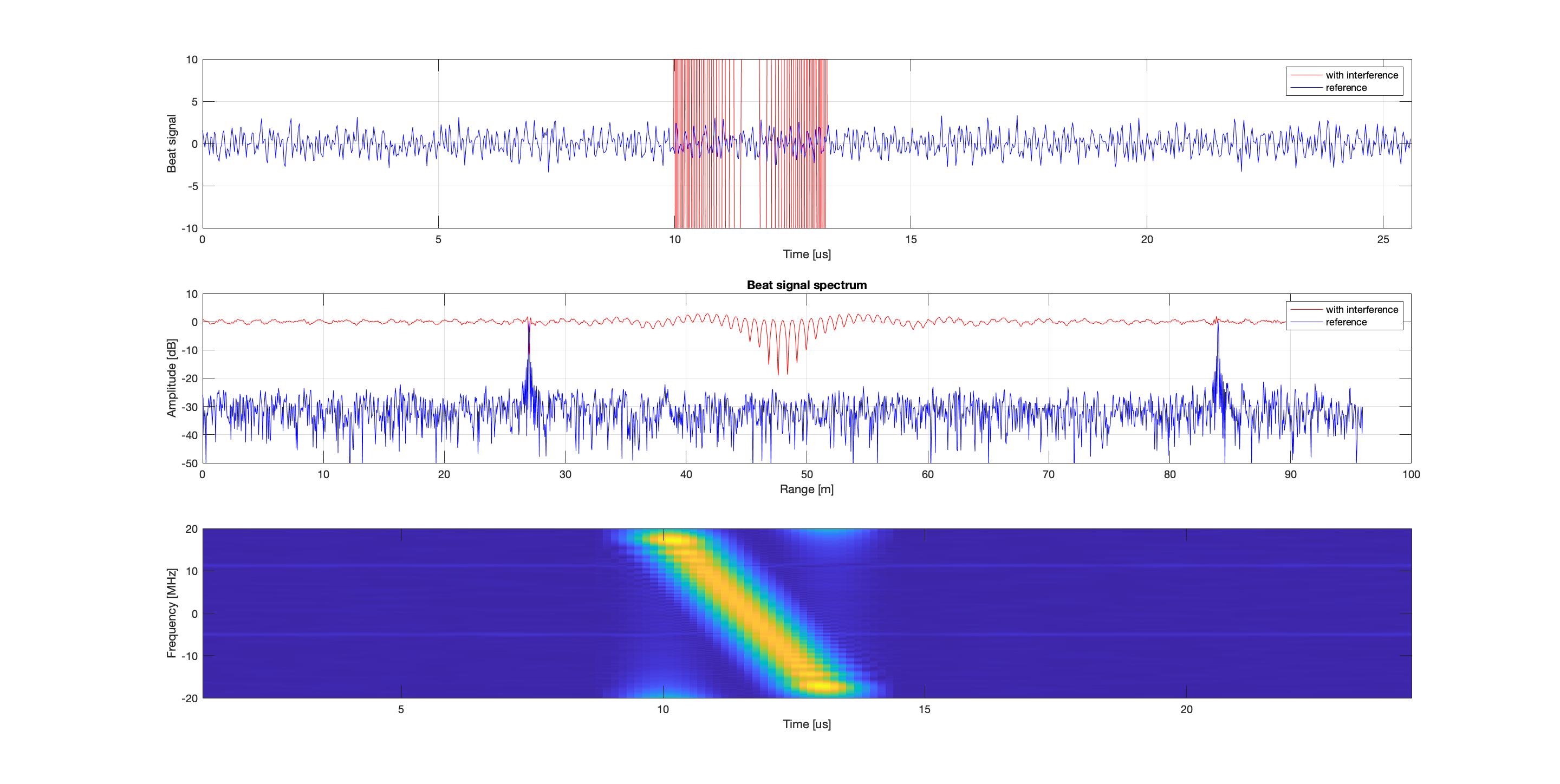
We also benchmark our proposed detector against a number of recently proposed multitarget CFAR detectors and show an improvement of 16% in probability of detection compared to censored harmonic averaging CFAR, with even larger improvements compared to both outlier-robust CFAR and truncated statistics log-normal CFAR in our particular indoor scenario. We experimentally show that our proposed algorithm drastically outperforms ordered statistics CFAR (OS-CFAR) (standard detector used in automotive systems) for our specific task of indoor drone navigation with more than 19% higher probability of detection for a given probability of false alarm. Inspired by the advances in nonlinear target detection, In this article, we propose a novel high performance, yet low-complexity target detector and we experimentally validate our algorithm on a dataset acquired using a radar mounted on a drone. Traditionally, most radar systems use conventional constant false alarm rate (CFAR) detectors but their performance degrades in indoor scenarios with many reflectors. Central to radar performance is the detection algorithm used to separate targets from the background noise and clutter. In those novel scenarios, radars must perform well in dense scenes with a large number of neighboring scatterers. The experiments show that the proposed method is capable to select properly based on the best performance of both CA and OS CFAR in homogeneous and non-homogeneous environments.Ībstract: As radar sensors are being miniaturized, there is a growing interest for using them in indoor sensing applications such as indoor drone obstacle avoidance. The method was examined with three simulated common radar cases including homogeneous background, multi target and clutter wall environment. That threshold will be compared to CA and OS CFAR to select the best final threshold. The pattern of those inputs will be classified and recognized by the neural network by the training to calculate the preliminary threshold. The input of neural network was as many as 3 consisted of CA and OS CFAR and Cell Under Test (CUT) value. The neural network used in this research is the Multi-Layer Perceptron (MLP) consisted of two hidden layers. This method uses one of between CA-CFAR and OS-CFAR as output threshold depends on the nearest value with the output of neural network. Abstract: This paper presents the switching CA/OS CFAR using neural network for improving the radar target detection in non-homogeneous environment.
